White Paper
The Promise of Adaptive Learning Technology
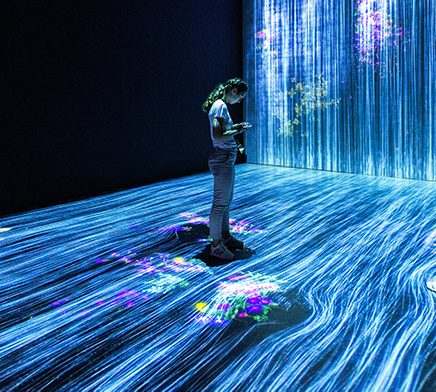
This white paper will explore one of the latest educational innovations that could prove to be revolutionary in advancing student-learning outcomes. This innovation is known as adaptive learning technology. We will first of all define its core principles and explore its philosophical goals. Once defined, we will take a look at one of the leading adaptive platforms that is gaining some traction in the educational technology space. We will continue to take a look at some of the current research about this platform and capture some of its core findings. After discussing some of the key merits we will also discuss some potential pitfalls. Lastly, we will explore some potential future developments based on adaptive learning technology.
What is adaptive learning?
Adaptive technology is designed to promote adaptive learning. Adaptive learning is learning that takes place in response to behavioral inputs. In other words, based on the user’s input the “program” that the user is interfacing with will adapt itself to the ability of the user to enable optimized learning. To use a simple example, if the user can’t provide the correct solution to a fairly simple multiplication problem the machine will adapt itself to the user’s input by not providing a more complex problem. In fact, the “program” should provide either a similar problem in terms of its level of difficulty or even a simpler problem and then enable a “scaffolded” learning experience such that the user can jump from one level to the next.
So how does all of this work?
It is clear there are three major components that need to be integrated to support an adaptive learning platform. First, the educational material needs to be sophisticated enough to support adaptivity. It has to be developed in a bookmarks folder “untitled folder”highly scaffolded manner in order to support the right kinds of learning levels. If the material is not developed at different levels, then it won’t be able to support thinkers who need more help to understand a particular concept or won’t advance an accelerated learner. In other words, it won’t be able to support differentiated learning. To reiterate, adaptivity requires more and more source data.
The implications of this last point are quite dramatic for content developers. What it entails is that content needs to be sliced and diced into smaller chunks. And this leads to the second component—metadata. Content needs to be tagged by appropriate metadata—especially when it comes to how to “weight” it in terms of difficulty. In other words, you could tag the “chunk” of content in terms of beginner, intermediate, or advanced levels. Some kind of assessment (also leveled) should also be tied to these units of content. Once you’ve sliced and diced the content, the content developer will then need to “position” the piece of content on the appropriate “branch” on the appropriate “learning tree”. The tree will represent various learning paths the user can take to enable knowledge and understanding of the appropriate course of study. You can think of the tree as a complete course of study or a complete book on a particular topic. The structure of the learning tree is not unlike a table of contents. The leaves on the branches represent the “chunks” of learning and might be based around a learning objective. As you acquire understanding of the particular objective, as measured via the associated assessment, you make progress down a particular branch of learning. And as you jump from branch to branch, you are traversing various learning paths that can be designed in non-linear ways that ultimately leads to mastery of the particular course of study. Now if this “tree” is adaptive in nature, then it may move the user around the tree as it adapts itself to the user’s input. So it may move the user to a previous leaf, or a prior branch, or to a higher branch, or to a branch that provides a modality of learning that supports learning preferences that the machine has already glimpsed based on existing input behavior.
The third component is the set of computer algorithms that apply to this educational material. If I get this question wrong, the algorithm needs to be able to “know” not to give me another question that is more complicated than the one I just got wrong. Also, in similar fashion to Amazon’s personalization engine, algorithms need to be structured to understand a user’s preference and make predictive choices based on user input. If I purchase a number of biographies from Amazon, then it should be smart enough to suggest similar kinds of books when I’m visiting the site. If I’m selecting a large number of horror movies from Netflix, then the platform should be smart enough to make horror recommendations when I’m navigating the site. Similarly, in the learning space, the system should be smart enough; for example, to know that based on my behavioral inputs it can predict that I’m a much better visual learner than analytical learner. The computer program should be responding to my answer to a quiz for a particular learning objective, to my mouse behavior, to the time it takes to click on something, to what I click on, and to a host of other inputs to create a personalized learning user profile. Based on that profiling, it can then predict my learning modality preferences.
An Adaptive Learning Platform
One of the leading players in the adaptive learning space is a company called Knewton. They make the point that there are multiple meanings of adaptive learning but that the most common usage of that term indicates a notion known as single-point adaptivity. What this means is similar to computer adaptive testing, where a user gets presented questions based on level of difficulty as a function of the presumed skill level of the user. Unlike this, the Knewton platform is “continuously adaptive”. In their words:
…we mean a system that is continuously adaptive — that responds in real-time to each individual’s performance and activity on the system and that maximizes the likelihood a student will obtain her learning objectives by providing the right instruction, at the right time, about the right thing. In other words, while adaptive testing answers the question, “How do I get the most accurate picture of a student’s state of knowledge with a fixed number of questions?”, adaptive learning answers the question, “Given what we understand about a student’s current knowledge, what should that student be working on right now?” (Knewton Whitepaper, http://www.knewton.com/adaptive-learning-white-paper/)
Based on data-mining techniques and algorithms applied to that usage data, the system makes learning recommendations based on what it learns about the user.
Key Findings
The goals of this platform are that it enables better learning outcomes, better engagement, and better retention for the user. So is there research data that supports this correlation? Is there a causal connection such that using the Knewton adaptive platform leads to better learning outcomes? They have recently conducted a pilot at Arizona State University with their Math Readiness Program:
Knewton Math Readiness, for example, creates a guided, self-paced environment in which live instruction is optimized around targeted group sessions. The course is designed to present students with personal learning paths as it continually assesses their mathematical proficiency and adapts accordingly. Lessons consist of videos, online textbook selections, and lesson quizzes. Early efficacy reports reflect the success of the program: after two semesters of use with over 2,000 developmental math students at Arizona State University, withdrawal rates dropped by 56%, pass rates went from 64% to 75%, and 45% of the class finished four weeks early. (Knewton Whitepaper, http://www.knewton.com/adaptive-learning-white-paper/)
So we see in the case of this pilot, the Knewton platform shows improved learning outcomes for more and more students, improved student retention rates as they work through this educational program, and improved efficiency around the completion rate of this educational program.
Different Directions
There are quite a few directions that adaptive learning can take and is taking. Gamification is one such direction. For example:
Alleyoop is betting that by combining adaptive learning with some elements of gamification, it can help prepare high schoolers and young people for college — and increase college graduation rates. (http://techcrunch.com/2012/02/01/pearson-incubated-startup-alleyoop-launches-to-gamify-adaptive-learning/)
You can see adaptivity built into a number of computer games already. The games often lead the user through different difficulty levels, where they gain mastery of a particular level before progressing to the next level. The “learning path” is clearly scaffolded. Games are fun, engaging, and suspenseful. If you can build these elements into an adaptive learning environment to promote student engagement and improved learning mastery, then this will be a valuable direction in which to go.
Learning analytics is another direction or perhaps layer on top of adaptive learning. It requires data mining, algorithmic structures that can measure performance, and the analytic engine can often measure student engagement with the object of their learning. Learning analytics allow teachers to address the needs of different students and also gain deeper insights into the learning proficiencies exhibited by their students.
Pros and Cons
We’ve looked at some of the benefits of adaptive learning already: supports personalized learning, differentiated learning, economy of learning, optimized learning, improved retention, improved learning, and increased engagement. But what are some drawbacks about adaptive learning.
A potential issue is related to the “big brother” phenomenon. In order to be adaptive, the system needs to know a lot about the user. It does a lot of data mining and builds personal profiles of its users. A system will often know more about the user than the user will know about itself. It may know how many pages you have read, how many notes/highlights/bookmarks you have made, what preferences you have about a particular topic, what your strengths and weaknesses might be. This can all be beneficial to the user. But on the flipside, big brother may not always play nice.
Related to this is the issue of personal privacy. Users want to protect their privacy and they don’t want their personal data to get into the hands of third-party advertising companies. However, adaptive engines need to know a lot about the user in order to be effective, so there is potential for conflict here.
Another issue might be the “dumbing down” of the learning experience as the user adapts to learning paths that suit their learning preferences. Maybe it’s a good thing for users to go down non-preferred learning paths. Maybe developing an individual’s analytical skills is a good thing, even if the preferred learning modality of the user is visual learning. Maybe learning geometry should not be as much fun or as engaging as a game of AngryBirds! So we might just be reinforcing the user’s strengths and not developing their weaknesses as well.
Summary
It is clear that adaptive learning could prove to be a game-changer in the educational learning space. If it can consistently be shown to improve learning outcomes for more and more students or improve student retention rates as they work through various educational programs, or improve more efficiency around completion of educational programs, then it will need to be taken very seriously by the educational community at large. If this can be achieved, while at the same time minimizing the potential pitfalls around this technological innovation, then we will bear witness to a tremendous educational breakthrough that could impact a majority of 21st century learners.
Related to this is the issue of personal privacy. Users want to protect their privacy and they don’t want their personal data to get into the hands of third-party advertising companies. However, adaptive engines need to know a lot about the user in order to be effective, so there is potential for conflict here.
Another issue might be the “dumbing down” of the learning experience as the user adapts to learning paths that suit their learning preferences. Maybe it’s a good thing for users to go down non-preferred learning paths. Maybe developing an individual’s analytical skills is a good thing, even if the preferred learning modality of the user is visual learning. Maybe learning geometry should not be as much fun or as engaging as a game of AngryBirds! So we might just be reinforcing the user’s strengths and not developing their weaknesses as well.
Jozefa Stefaniak
April 4, 2013
Need to see more samples of my work?
Let's Get Started
- Articulate Storyline
- Camtasia
- InDesign
- Adobe Captivate
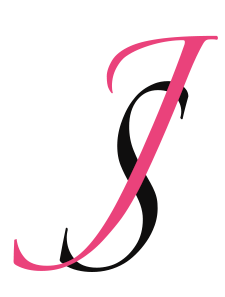